Life & Earth - Deep Transfer Learning
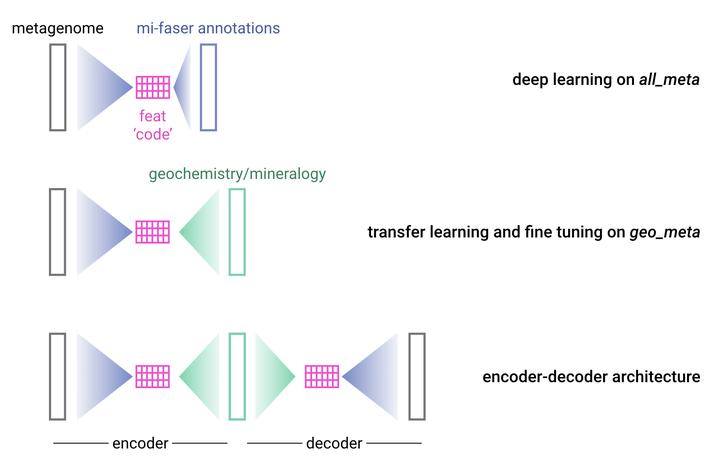
Creating functional maps of protein sequences
The network of interactions linking the biosphere and the geosphere is vastly complex. This complexity obscures the biogeochemically relevant features underlying the noisy and diverse microbial communities inhabiting our planet. It thus presents both a significant challenge and exciting opportunity to apply new computational approaches to modeling microbial interactions with the Earth system. Deep learning techniques are ideal for high complexity systems. Here I propose to train a deep neural network on all publicly available metagenomes to learn the complex features underlying microbial communities, and using transfer learning, to leverage these features to link environmental microbes to their associated geochemistry and mineralogy. These models will also allow me to validate the presence of key protein motifs under variable geochemical regimes, under both modern and ancient Earth conditions.
To this end, I will introduce a novel data augmentation approach to produce the millions of metagenomic inputs necessary for deep learning to be most effective. I will train the first deep learning model on all publicly available metagenomes, deriving those functionally relevant features underlying all microbial communities. Using transfer learning, I will leverage the complex features learned on this metagenome corpus to predict geochemical and mineralogical compositions from a smaller curated set of metagenomes with known mineralogy and geochemistry. This effort will create models that capture and predict the complexity of the bio-geosphere at a depth that is currently intractable.